Peering Into The Black Box of Large Language Models
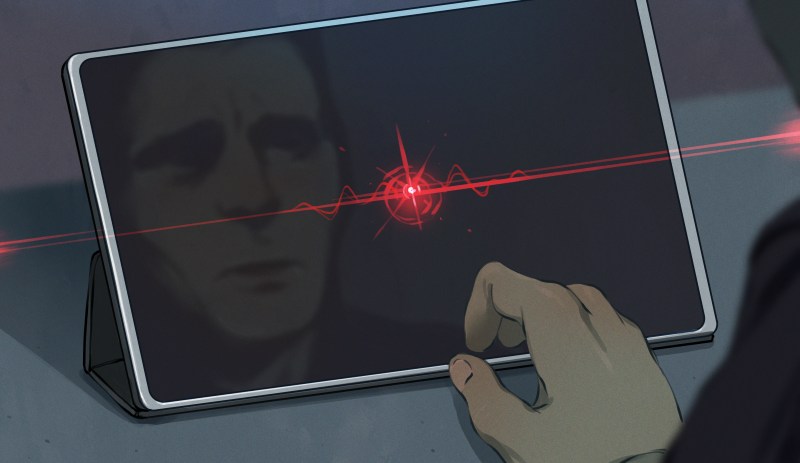
Large Language Models (LLMs) can produce extremely human-like communication, but their inner workings are something of a mystery. Not a mystery in the sense that we don’t know how an LLM works, but a mystery in the sense that the exact process of turning a particular input into a particular output is something of a black box.
This “black box” trait is common to neural networks in general, and LLMs are very deep neural networks. It is not really possible to explain precisely why a specific input produces a particular output, and not something else.
Why? Because neural networks are neither databases, nor lookup tables. In a neural network, discrete activation of neurons cannot be meaningfully mapped to specific concepts or words. The connections are complex, numerous, and multidimensional to the point that trying to tease out their relationships in any straightforward way simply does not make sense.
Neural Networks are a Black Box
In a way, this shouldn’t be surprising. After all, the entire umbrella of “AI” is about using software to solve the sorts of problems humans are in general not good at figuring out how to write a program to solve. It’s maybe no wonder that the end product has some level of inscrutability.
This isn’t what most of us expect from software, but as humans we can relate to the black box aspect more than we might realize. Take, for example, the process of elegantly translating a phrase from one language to another.
I’d like to use as an example of this an idea from an article by Lance Fortnow in Quanta magazine about the ubiquity of computation in our world. Lance asks us to imagine a woman named Sophie who grew up speaking French and English and works as a translator. Sophie can easily take any English text and produce a sentence of equivalent meaning in French. Sophie’s brain follows some kind of process to perform this conversion, but Sophie likely doesn’t understand the entire process. She might not even think of it as a process at all. It’s something that just happens. Sophie, like most of us, is intimately familiar with black box functionality.
The difference is that while many of us (perhaps grudgingly) accept this aspect of our own existence, we are understandably dissatisfied with it as a feature of our software. New research has made progress towards changing this.
Identifying Conceptual Features in Language Models
We know perfectly well how LLMs work, but that doesn’t help us pick apart individual transactions. Opening the black box while it’s working yields only a mess of discrete neural activations that cannot be meaningfully mapped to particular concepts, words, or whatever else. Until now, that is.
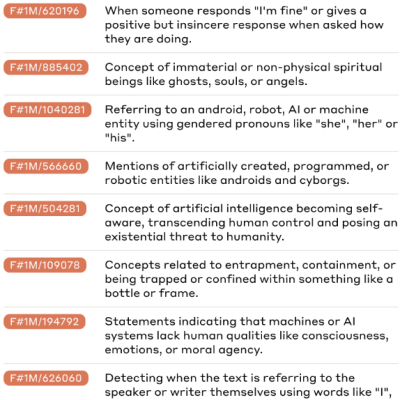
Recent developments have made the black box much less opaque, thanks to tools that can map and visualize LLM internal states during computation. This creates a conceptual snapshot of what the LLM is — for lack of a better term — thinking in the process of putting together its response to a prompt.
Anthropic have recently shared details on their success in mapping the mind of their Claude 3.0 Sonnet model by finding a way to match patterns of neuron activations to concrete, human-understandable concepts called features.
A feature can be just about anything; a person, a place, an object, or more abstract things like the idea of upper case, or function calls. The existence of a feature being activated does not mean it factors directly into the output, but it does mean it played some role in the road the output took.
With a way to map groups of activations to features — a significant engineering challenge — one can meaningfully interpret the contents of the black box. It is also possible to measure a sort of relational “distance” between features, and therefore get an even better idea of what a given state of neural activation represents in conceptual terms.
Making Sense of it all
One way this can be used is to produce a heat map that highlights how heavily different features were involved in Claude’s responses. Artificially manipulating the weighting of different concepts changes Claude’s responses in predictable ways (video), demonstrating that the features are indeed reasonably accurate representations of the LLM’s internal state. More details on this process are available in the paper Scaling Monosemanticity: Extracting Interpretable Features from Claude 3 Sonnet.
Mapping the mind of a state-of-the-art LLM like Claude may be a nontrivial undertaking, but that doesn’t mean the process is entirely the domain of tech companies with loads of resources. Inspectus by [labml.ai] is a visualization tool that works similarly to provide insight into the behavior of LLMs during processing. There is a tutorial on using it with a GPT-2 model, but don’t let that turn you off. GPT-2 may be older, but it is still relevant.
Research like this offers new ways to understand (and potentially manipulate, or fine-tune) these powerful tools., making LLMs more transparent and more useful, especially in applications where lack of operational clarity is hard to accept.