An Animated Walkthrough of How Large Language Models Work
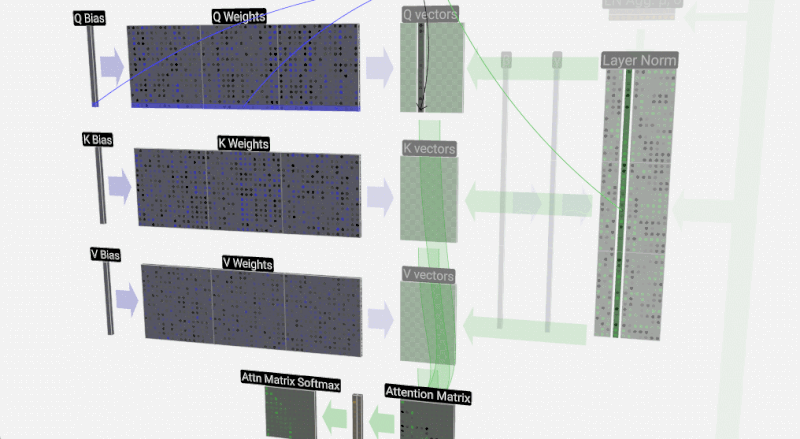
If you wonder how Large Language Models (LLMs) work and aren’t afraid of getting a bit technical, don’t miss [Brendan Bycroft]’s LLM Visualization. It is an interactively-animated step-by-step walk-through of a GPT large language model complete with animated and interactive 3D block diagram of everything going on under the hood. Check it out!
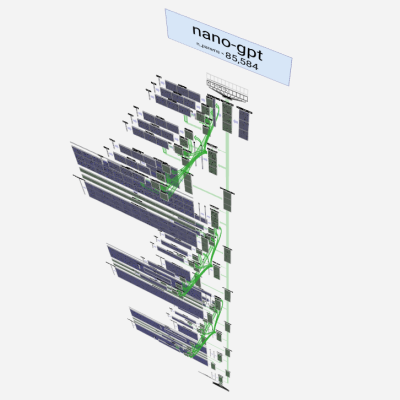
The demonstration walks through a simple task and shows every step. The task is this: using the nano-gpt model, take a sequence of six letters and put them into alphabetical order.
A GPT model is a highly complex prediction engine, so the whole process begins with tokenizing the input (breaking up words and assigning numerical values to the chunks) and ends with choosing an appropriate output from a list of probabilities. There are of course many more steps in between, and different ways to adjust the model’s behavior. All of these are made quite clear by [Brendan]’s process breakdown.
We’ve previously covered how LLMs work, explained without math which eschews gritty technical details in favor of focusing on functionality, but it’s also nice to see an approach like this one, which embraces the technical elements of exactly what is going on.
We’ve also seen a much higher-level peek at how a modern AI model like Anthropic’s Claude works when it processes requests, extracting human-understandable concepts that illustrate what’s going on under the hood.